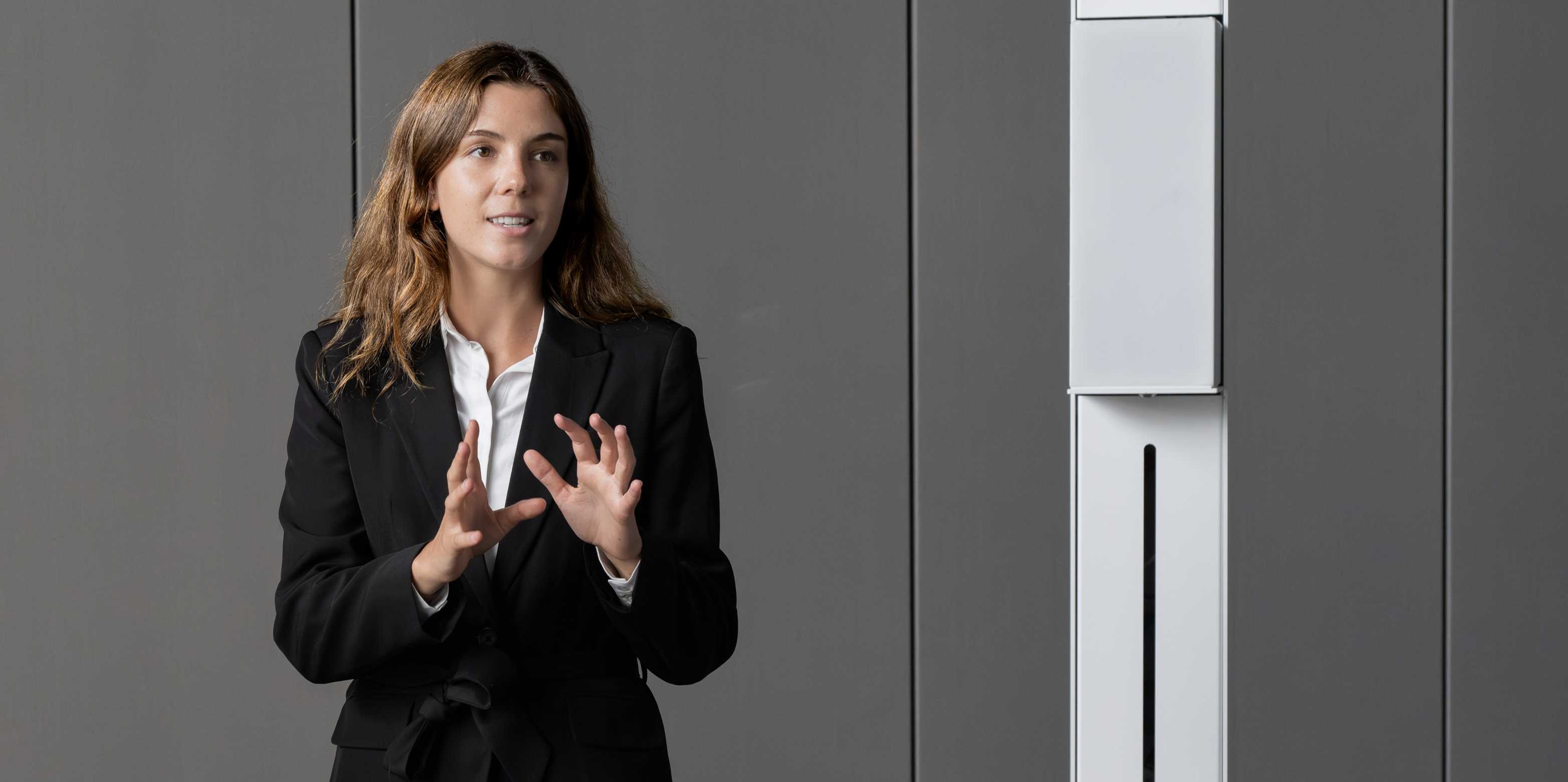
"I use data-driven methods to enable real-time structural assessments, which can minimize costly and resource-intensive replacements of aging infrastructure."
Sophia Kuhn is pursuing her PhD under Prof. Dr. Walter Kaufmann at ETH Zurich. Her research focuses on integrating AI and machine learning into bridge design and infrastructure assessment to improve efficiency and sustainability.
Can you describe your doctoral research project?
My research focuses on harnessing Artificial Intelligence (AI), specifically Machine Learning (ML) algorithms, to assist structural engineers in designing efficient bridge structures and in assessing existing bridges to conserve resources.
When designing new bridge structures, engineers face the complex task of developing a suitable design that complies with the project’s unique boundary conditions, safety and serviceability requirements and budget constraints. Current software tools can only evaluate designs after the engineer has come up with a suggestion for the numerous interrelated design variables. This makes the design development a lengthy, iterative process that often leaves a lot of possibilities and, therefore, potential unexplored.
To address this challenge, I develop generative AI models that act as design co-pilots, proactively suggesting efficient design solutions that meet defined constraints and objectives (cf. Image 1). Specifically, we developed a project-tailored co-pilot for the design of a pedestrian bridge in St. Gallen, Switzerland (Balmer and Kuhn et al., 2024), as well as a more broadly applicable co-pilot for the design of concrete frame bridge structures (Kuhn et al., 2023) (cf. Image 2). These studies show that the trained deep learning models suggest designs that comply with project constraints and objectives (including structural performance, environmental impact metrics, and cost) reducing the number of necessary design iterations and also significantly accelerate the evaluation process.
Additionally, I apply data-driven methods as a real-time assessment tool for existing structures, helping to extend their lifespan and reduce necessary resource-intensive strengthening or replacement measures.


What inspired your research?
The Architecture, Engineering, and Construction (AEC) industry is at a pivotal moment where traditional methods can no longer meet the efficiency and sustainability demands of today, especially given the significant environmental impact of the construction sector. I believe data-driven methods, which are currently underutilized in AEC, are key drivers of this necessary change. However, this approach requires the development of large-scale, high-quality, and well-structured AEC data repositories. I see great potential in combining real-world data with simulation databases. In our current application studies, we rely heavily on parametric simulation pipelines to generate bridge-type-specific data, but we are actively working on gathering, structuring, and incorporating real-world data as well. My passion lies in combining intelligent algorithms with real-world problems to develop innovative, economical, and sustainable solutions.
What are the potential real-world applications of your research within the AEC industry? How could it impact everyday life?
In collaboration with a team of interdisciplinary researchers from Kaufmann research group, the Swiss Data Science Center and Gramazio Kohler Research we have developed the open-source AI-eXtended Design Toolbox (AIXD) to bring data-driven methodologies into the AEC sector. The toolbox provides advanced machine learning (ML) techniques through simple, high-level commands, making these powerful tools accessible to domain experts like civil engineers and architects. The goal is to facilitate collaborative design between the users and AI, fostering more efficient design solutions. Though initially developed for architectural and engineering design, AIXD’s problem-agnostic approach makes it applicable to various domains beyond AEC.
ML methods are not only beneficial for designing new structures but also offer advantages in managing the large stock of existing structures. In Switzerland and many other countries (i.a. Germany, Austria and the USA), a significant number of bridge structures of the national road and rail network approach their expected lifespan. Therefore, a critical challenge arises for maintaining the infrastructure network. Our newly developed ML prediction model for structural assessment aims to help prioritise which structures need immediate attention (cf. Image 3). The goal is to ensure that appropriate measures are taken for the right structures at the right time, benefiting asset owners' budgets and reducing the environmental impact of unnecessary strengthening or replacement of structures.


Can you please share a surprising or unexpected outcome you have encountered during your research so far?
What has surprised me is the significant untapped potential in the assessment and management of existing infrastructure, which is especially crucial in Switzerland today. Data-driven methods combined with the necessary data can provide more standardized and objective approaches to evaluating and managing our ageing infrastructure. Our models have proven valuable in pinpointing which structures have substantial structural reserves, enabling the reduction of necessary strengthening measures with advanced structural assessment methods, such as non-linear Finite Element analysis. We are working together with the SBB AG to leverage this tool in the management of Swiss railway bridge structures, aiming to extend their lifespan and conserve resources.
What is augmented computational design for you?
For me, augmented computational design is about enhancing human capabilities by combining the strengths of machines with those of human designers and engineers. Machines excel at performing complex calculations and processing large amounts of data quickly, while humans are often better at seeing the bigger picture and understanding the individuality of each project as well as the context.
References:
- external page Balmer and Kuhn et al., 2024, Design Space Exploration and Explanation via Conditional Variational Autoencoders in Meta-Model-Based Conceptual Design of Pedestrian Bridges.
- Kuhn et al., 2023, Assessment and Integration of Sustainability and Circularity Metricswithin Generative Bridge Design.
